Nonlinear Model Predictive Control with C-GMRES
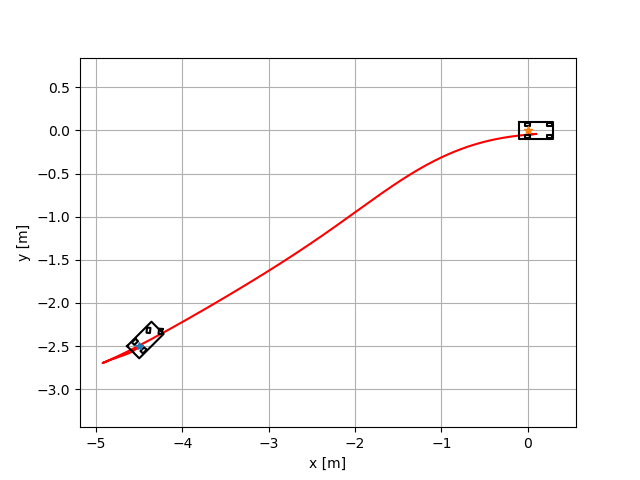
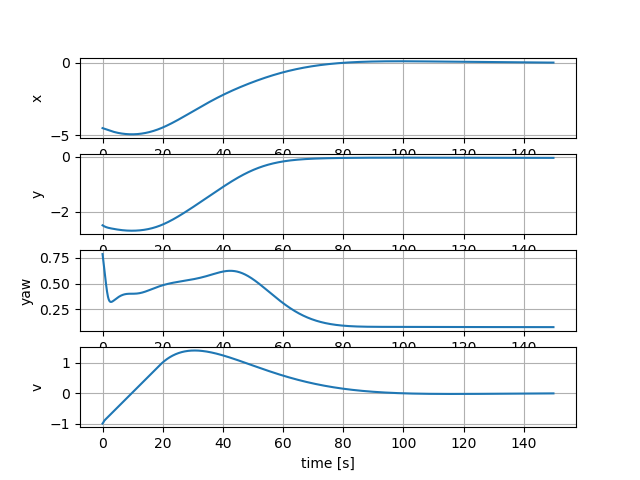
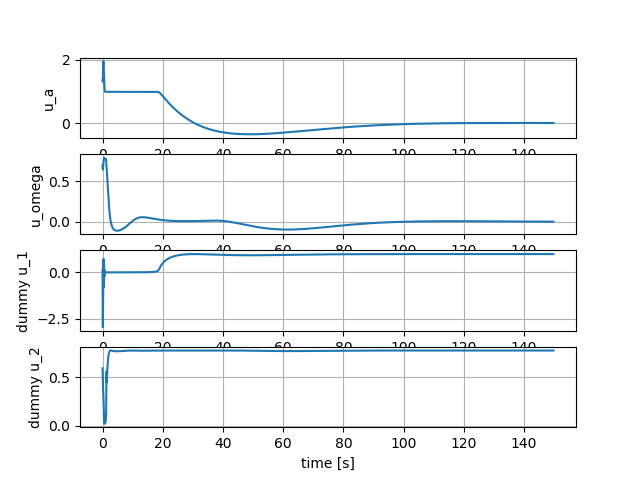
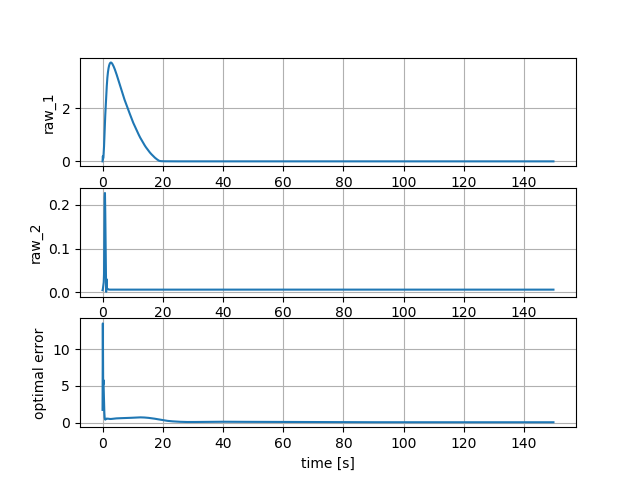

Code Link
- PathTracking.cgmres_nmpc.cgmres_nmpc.NMPCControllerCGMRES()[source]
- PathTracking.cgmres_nmpc.cgmres_nmpc.zeta
gain of optimal answer stability
- Type:
float
- PathTracking.cgmres_nmpc.cgmres_nmpc.ht
update value of NMPC this should be decided by zeta
- Type:
float
- PathTracking.cgmres_nmpc.cgmres_nmpc.tf
predict time
- Type:
float
- PathTracking.cgmres_nmpc.cgmres_nmpc.alpha
gain of predict time
- Type:
float
- PathTracking.cgmres_nmpc.cgmres_nmpc.N
predict step, discrete value
- Type:
int
- PathTracking.cgmres_nmpc.cgmres_nmpc.threshold
cgmres’s threshold value
- Type:
float
- PathTracking.cgmres_nmpc.cgmres_nmpc.input_num
system input length, this should include dummy u and constraint variables
- Type:
int
- PathTracking.cgmres_nmpc.cgmres_nmpc.max_iteration
decide by the solved matrix size
- Type:
int
- PathTracking.cgmres_nmpc.cgmres_nmpc.simulator
- Type:
NMPCSimulatorSystem class
- PathTracking.cgmres_nmpc.cgmres_nmpc.u_1s
estimated optimal system input
- Type:
list of float
- PathTracking.cgmres_nmpc.cgmres_nmpc.u_2s
estimated optimal system input
- Type:
list of float
- PathTracking.cgmres_nmpc.cgmres_nmpc.dummy_u_1s
estimated dummy input
- Type:
list of float
- PathTracking.cgmres_nmpc.cgmres_nmpc.dummy_u_2s
estimated dummy input
- Type:
list of float
- PathTracking.cgmres_nmpc.cgmres_nmpc.raw_1s
estimated constraint variable
- Type:
list of float
- PathTracking.cgmres_nmpc.cgmres_nmpc.raw_2s
estimated constraint variable
- Type:
list of float
- PathTracking.cgmres_nmpc.cgmres_nmpc.history_u_1
time history of actual system input
- Type:
list of float
- PathTracking.cgmres_nmpc.cgmres_nmpc.history_u_2
time history of actual system input
- Type:
list of float
- PathTracking.cgmres_nmpc.cgmres_nmpc.history_dummy_u_1
time history of actual dummy u_1
- Type:
list of float
- PathTracking.cgmres_nmpc.cgmres_nmpc.history_dummy_u_2
time history of actual dummy u_2
- Type:
list of float
- PathTracking.cgmres_nmpc.cgmres_nmpc.history_raw_1
time history of actual raw_1
- Type:
list of float
- PathTracking.cgmres_nmpc.cgmres_nmpc.history_raw_2
time history of actual raw_2
- Type:
list of float
- PathTracking.cgmres_nmpc.cgmres_nmpc.history_f
time history of error of optimal
- Type:
list of float
Mathematical Formulation
Motion model is
(tan is not good for optimization)
Cost function is
Input constraints are
So, Hamiltonian is
Partial differential equations of the Hamiltonian are:
\(\begin{equation*} \frac{\partial H}{\partial \bf{x}}=\\ \begin{bmatrix} \frac{\partial H}{\partial x}= 0&\\ \frac{\partial H}{\partial y}= 0&\\ \frac{\partial H}{\partial \theta}= -\lambda_1vsin\theta+\lambda_2vcos\theta&\\ \frac{\partial H}{\partial v}=-\lambda_1cos\theta+\lambda_2sin\theta+\lambda_3\frac{1}{WB}sin(u_{\delta})&\\ \end{bmatrix} \\ \end{equation*}\)
\(\begin{equation*} \frac{\partial H}{\partial \bf{u}}=\\ \begin{bmatrix} \frac{\partial H}{\partial u_a}= u_a+\lambda_4+2\rho_1u_a&\\ \frac{\partial H}{\partial u_\delta}= u_\delta+\lambda_3\frac{v}{WB}cos(u_{\delta})+2\rho_2u_\delta&\\ \frac{\partial H}{\partial d_a}= -\phi_a+2\rho_1d_a&\\ \frac{\partial H}{\partial d_\delta}=-\phi_\delta+2\rho_2d_\delta&\\ \end{bmatrix} \\ \end{equation*}\)